Table Of Content
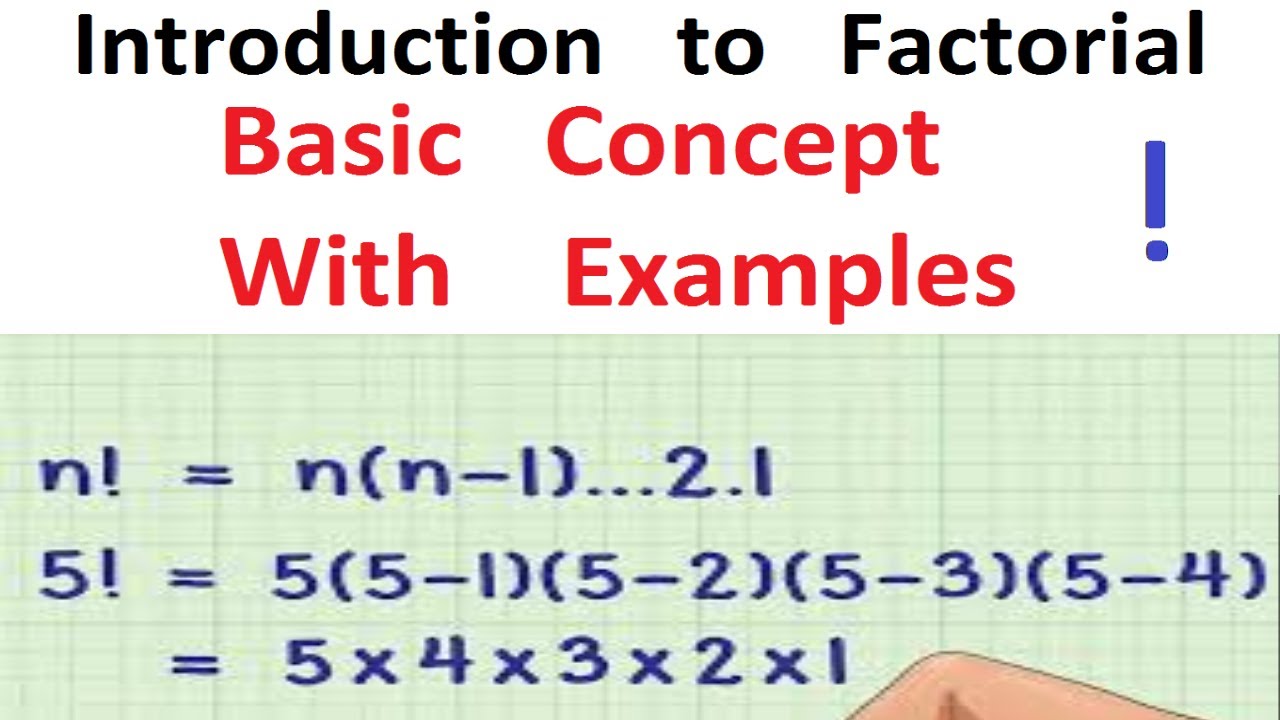
However the other two combinations, A and C and A and D, indicate that significant interaction exists. If you just looked at the main effects plot you would likely miss the interactions that are obvious here. You would find these types of designs used where k is very large or the process, for instance, is very expensive or takes a long time to run. In these cases, for the purpose of saving time or money, we want to run a screening experiment with as few observations as possible.
1 - The Simplest Case
For example, the entries in the B column follow the same pattern as the middle component of "cell", as can be seen by sorting on B. There are various traditions to denote the levels of each factor. For example, a shrimp aquaculture experiment[9] might have factors temperature at 25°C and 35°C, density at 80 or 160 shrimp/40 liters, and salinity at 10%, 25% and 40%. In many cases, though, the factor levels are simply categories, and the coding of levels is somewhat arbitrary.
Factorial Designs¶
The traditional rules of the scientific method are still in force, so statistics require that every experiment be conducted in triplicate. This example was constructed so that the marginal means and the overall means are the same as in Example 1. The IV would be Mindset, with the levels being growth mindset or fixed mindset. You have been employed by SuperGym, a local personal training gym, who want an engineer's perspective on how to offer the best plans to their clients. SuperGym currently categorizes her clients into 4 body types to help plan for the best possible program.
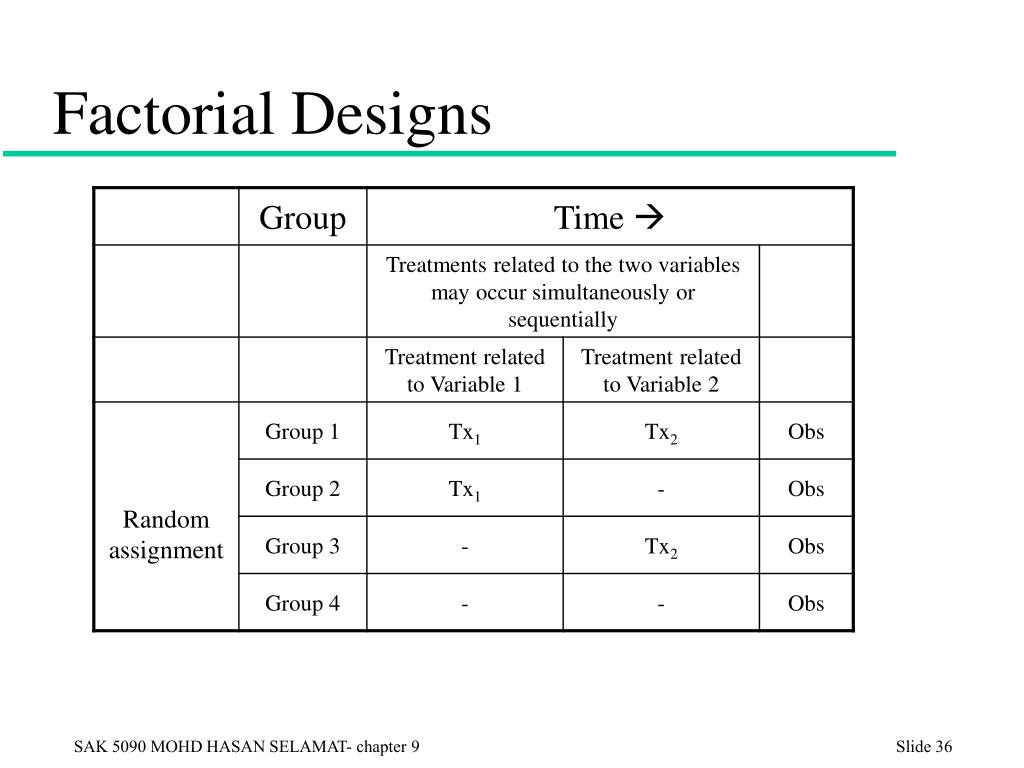
Basic Elements of RCT and Factorial Designs
Two additional points about factor analysis are worth making here. Factor analysis does not tell us that people are either extraverted or conscientious or that they like either “reflective and complex” music or “intense and rebellious” music. Instead, factors are constructs that operate independently of each other. So people who are high in extraversion might be high or low in conscientiousness, and people who like reflective and complex music might or might not also like intense and rebellious music. The second point is that factor analysis reveals only the underlying structure of the variables.
3.7. Interactions¶
The factorial design, as well as simplifying the process and making research cheaper, allows many levels of analysis. As well as highlighting the relationships between variables, it also allows the effects of manipulating a single variable to be isolated and analyzed singly. These equations can be used as a predictive model to determine wt% methanol in biodiesel and number of theoretical stages achieved at different operating conditions without actually performing the experiments.
3.5. Identifying main effects and interactions¶
However, when there is no interaction then we can remove the interaction terms from the model and use the reduced additive model. This example simply illustrates that the cell means, in this case, have additive structure. A problem with data that we actually look at is that you do not know in advance whether the effects are additive or not. Because of random error, the interaction terms are seldom exactly zero. You may be involved in a situation that is either additive or non-additive, and the first task is to decide between them. You might be wondering how this scenario on distractions and Spotting the Difference is related to factorial designs and ANOVAs since a t-test would be great at analyzing this data.
Experiment Design Guidelines for Product Analysts — Part 2/3 - ResearchGate
Experiment Design Guidelines for Product Analysts — Part 2/3.
Posted: Mon, 12 Jul 2021 07:00:00 GMT [source]
In the aquaculture experiment, the ordered triple (25, 80, 10) represents the treatment combination having the lowest level of each factor. In a general 2×3 experiment the ordered pair (2, 1) would indicate the cell in which factor A is at level 2 and factor B at level 1. The parentheses are often dropped, as shown in the accompanying table.
Interaction Effects
When we find that independent variable did influence the dependent variable, then we say there was a main effect. When we find that the independent variable did not influence the dependent variable, then we say there was no main effect. When researchers combine dependent variables in this way, they are treating them collectively as a multiple-response measure of a single construct. The advantage of this is that multiple-response measures are generally more reliable than single-response measures. However, it is important to make sure the individual dependent variables are correlated with each other by computing an internal consistency measure such as Cronbach’s \(\alpha\).
2.5. Graphing the Results of Factorial Experiments¶
In any case, your mom has to consider both the fertilizer type and amount of water provided to the plants when determining the proper growing conditions. From this table, we can see that there is positive correlation for factors A and C, meaning that more sleep and more studying leads to a better test grade in the class. Factor B, however, has a negative effect, which means that spending time with your significant other leads to a worse test score.
If the interaction is significant then we want to estimate and focus our attention on the cell means. If the interaction is not significant, then we can test the main effects and focus on the main effect means. There is really no benefit to the effects model when there is interaction, except that it gives us a mechanism for partitioning the variation due to the two treatments and their interactions.
This can be conducted with or without replication, depending on its intended purpose and available resources. It will provide the effects of the three independent variables on the dependent variable and possible interactions. The number of digits tells you how many independent variables (IVs) there are in an experiment, while the value of each number tells you how many levels there are for each independent variable. However, let’s imagine that she is also interested in learning if sleep deprivation impacts the driving abilities of men and women differently.
She has just added a second independent variable of interest (sex of the driver) into her study, which now makes it a factorial design. Again, because neither independent variable in this example was manipulated, it is a cross-sectional study rather than an experiment. But the two 2-way interactions effects combined are no longer significant, and individually, the interactions are not significant here either. So, the log transformation which improved the unequal variances pulled the higher responses down more than the lower values and therefore resulted in more of a parallel shape. Now we are in a position where we can drop the interactions and reduce this model to a main effects only model.
If that is true, its effects cannot be interpreted in a straightforward manner. If no adherence main effect is found, is that because this component was inconsistently delivered (adjusted for each medication)? In sum, investigators should be cognizant of the possible effects of such intervention adjustment and consider options for addressing them (e.g., by making only essential adjustments to a component, nesting an adjusted factor in the design).
No comments:
Post a Comment